The Multi-Modal Edge in Video Understanding with Anoki ContextIQ
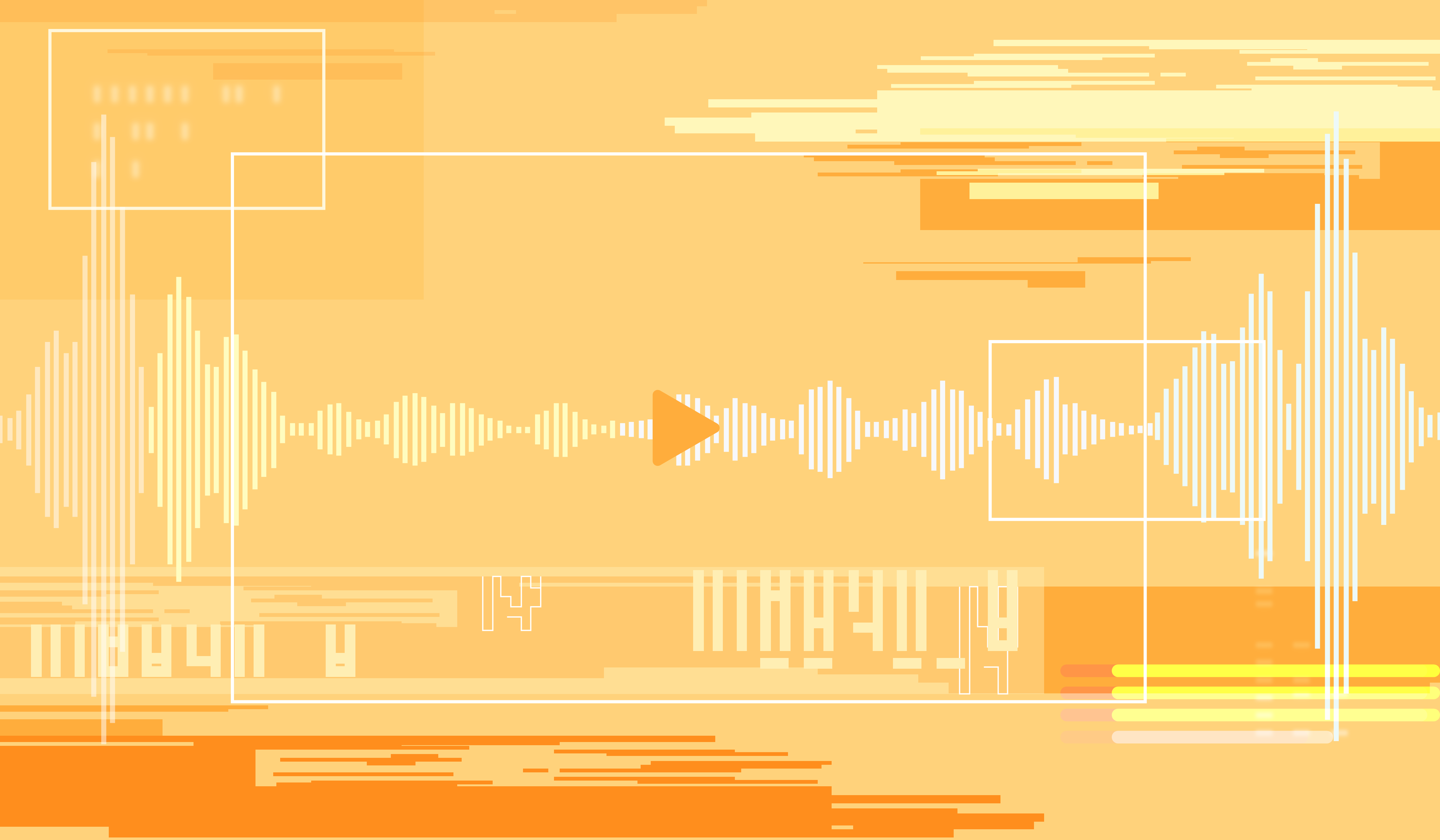
Introduction
Traditional video retrieval methods, which rely on isolated dimensions like frames, text transcripts, or object detection, often fail to capture the full narrative of a video. Video content is inherently complex, integrating visuals, dialogue, background sounds, and emotional cues that collectively shape its meaning.
Consider a beverage company promoting a summer drink—while a video-only approach might identify sunlit backyard scenes, it could miss the upbeat music that reinforces the mood or risk overlooking content that truly aligns with their brand’s message and seasonal theme.
To address this, Anoki’s ContextIQ system takes a multimodal approach, combining video, captions, audio, and enriched metadata to achieve a more comprehensive understanding of video content. By analyzing scenes across multiple pathways, ContextIQ mimics human-like perception and understanding, enabling more precise and effective ad placements.
In this blog, we explore how leveraging multiple modalities enhances both retrieval accuracy—ensuring the most relevant content is surfaced—and retrieval coverage—broadening the range of videos that align with diverse contextual needs.
Incremental Impact of Each Modality
Anoki’s ContextIQ system taps into multiple modalities; video, captions, audio, and other metadata extracted from the video such as objects, locations, entity presence, presence of profanity, and implicit/explicit hate speech and so on to create a richer, more holistic view of each piece of content/scene. Each modality offers unique insights that, when combined, significantly boost retrieval accuracy and coverage.
Retrieval Accuracy
Figure 1 compares video-only retrieval with retrieval augmented by additional modalities. Integrating captions, metadata, and audio consistently enhances precision across various Top-K values. On average, captions improve relative precision by 7.6%, metadata by 5.3%, and audio signals add another 6.2%, demonstrating the significant impact of multimodal integration.
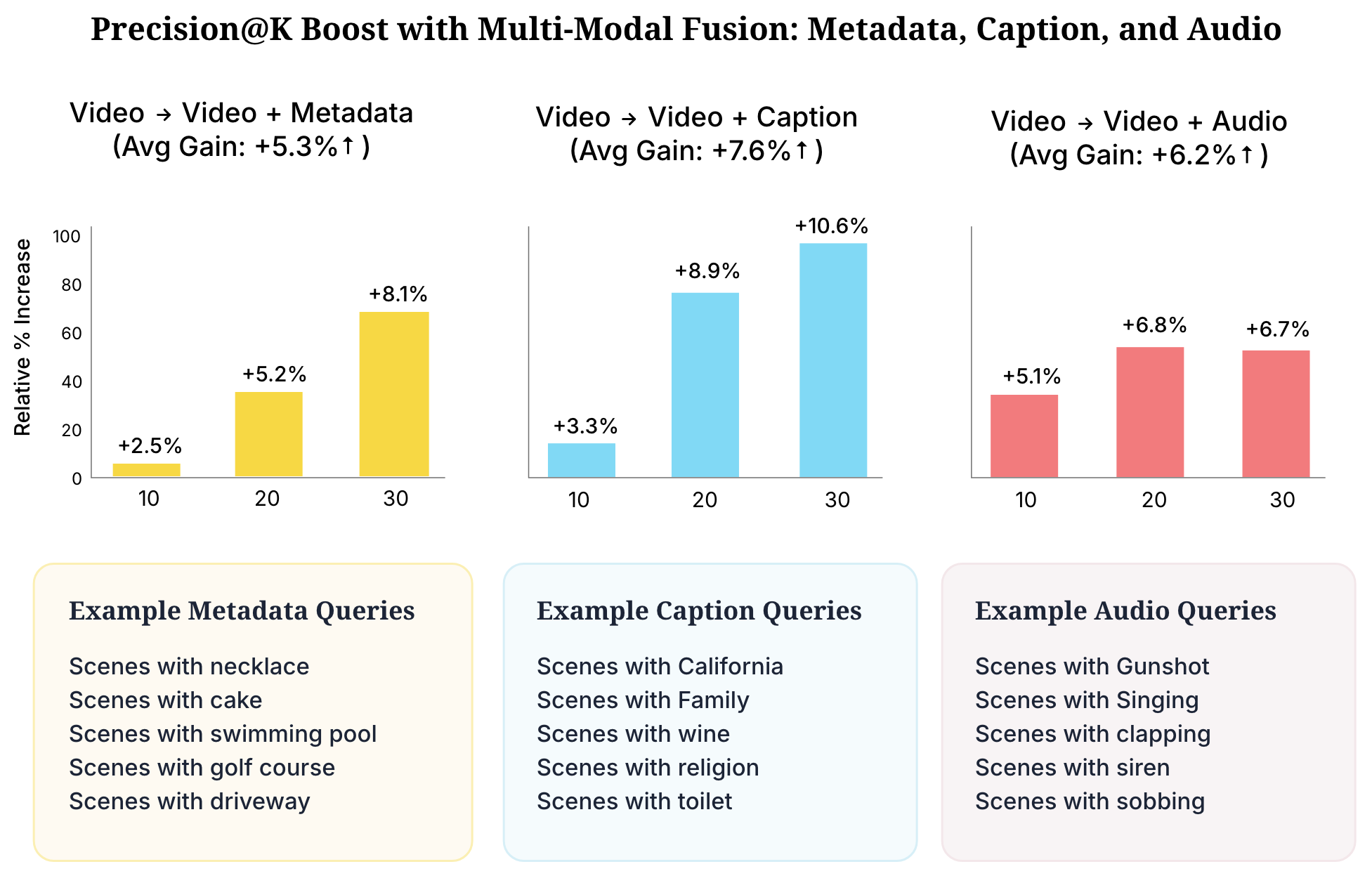
For each query, we measured the overlap between top results retrieved by different modalities at various K values. Figure 2 shows the overlap fractions of metadata, captions, and audio with the video modality at Top-K = 30. Lower overlap between modalities indicate that they capture distinct relevance cues, highlighting the importance of multimodal integration for broader coverage and improved overall performance.
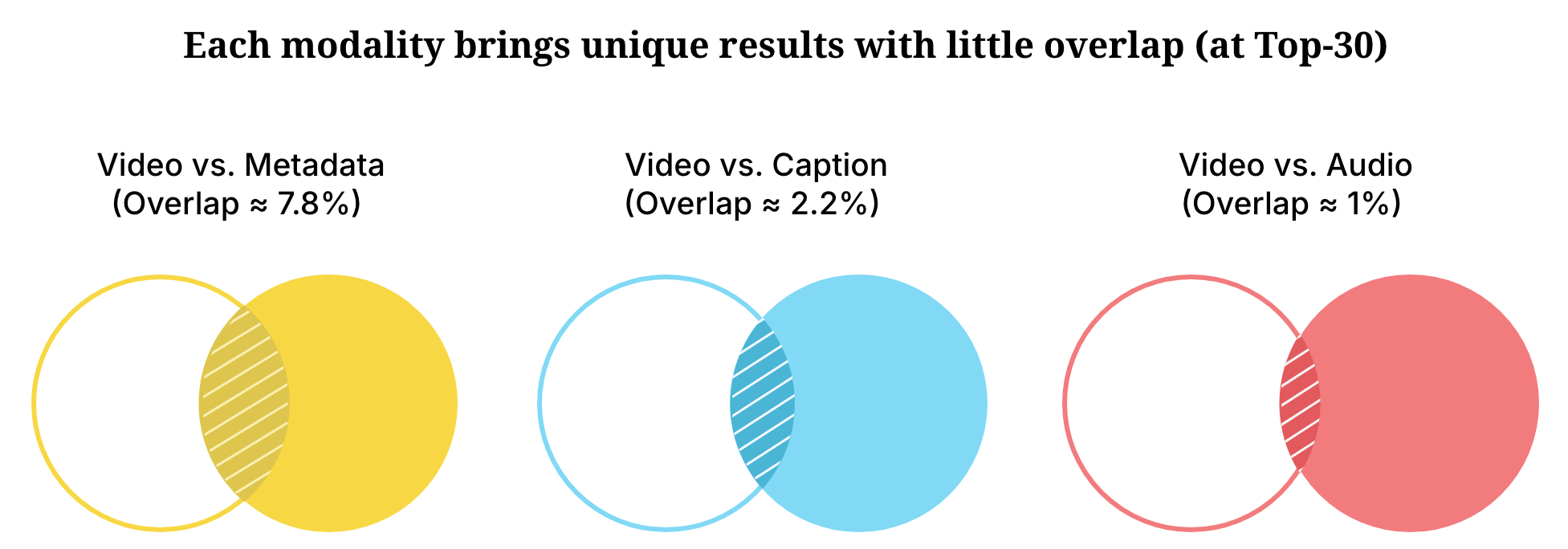
Retrieval Coverage
Beyond precision, understanding each modality's role in retrieving relevant content is crucial. Figure 3 illustrates the proportion of retrieved videos attributed to metadata across different queries. Queries such as "Scenes with guitars" or "Scenes with laptops" benefit significantly from object metadata, whereas “Scenes of museums” obviously gets a coverage boost from Locations metadata.
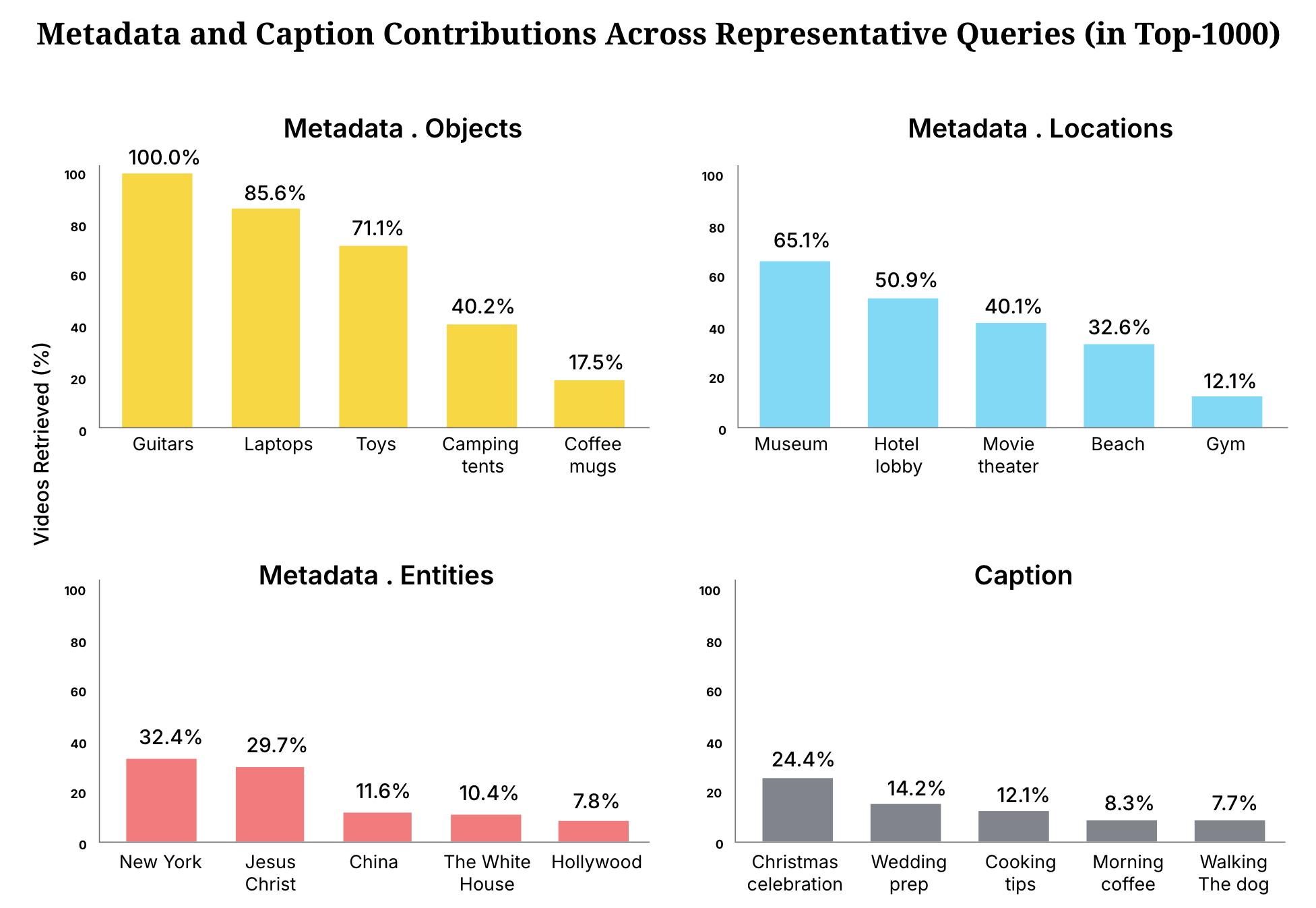
Conclusion
Integrating video, captions, metadata, and audio enables a deeper, human-like understanding of video content. As the data demonstrates, leveraging multiple modalities significantly enhances accuracy and coverage and makes Anoki ContextIQ uniquely positioned to deliver highly precise contextual advertising for video content.
Qualitative Example Videos
To showcase Anoki ContextIQ's multimodal capabilities, here are four qualitative examples — each demonstrating how a specific modality excels in retrieving relevant content.
Metadata
Query: Scenes with a wine glass
Retrieved Result by Metadata Modality:
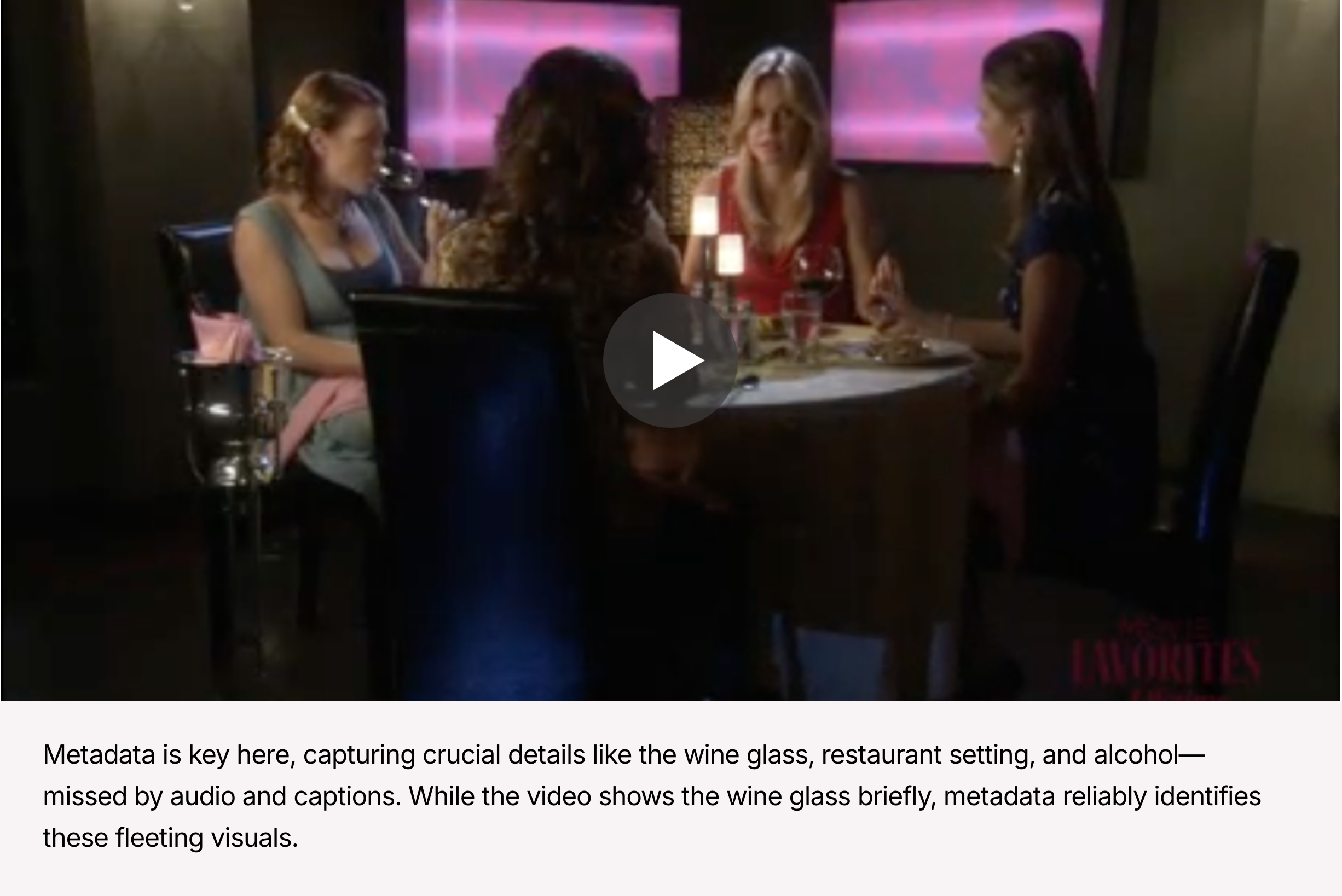
Caption
Query: Scenes with California
Retrieved Result by Caption Modality:
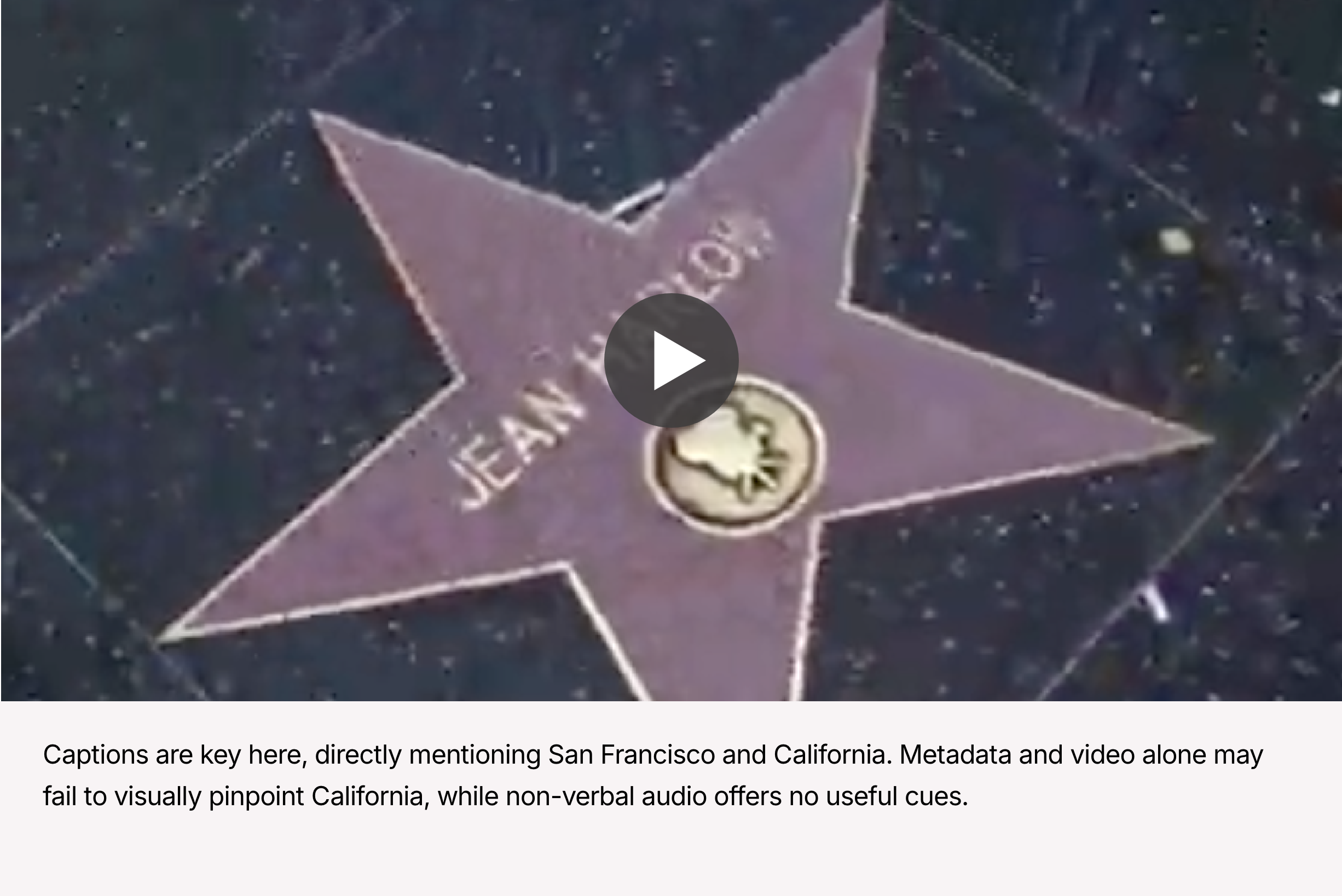
Audio
Query: Scenes with Singing
Retrieved Result by Audio Modality:
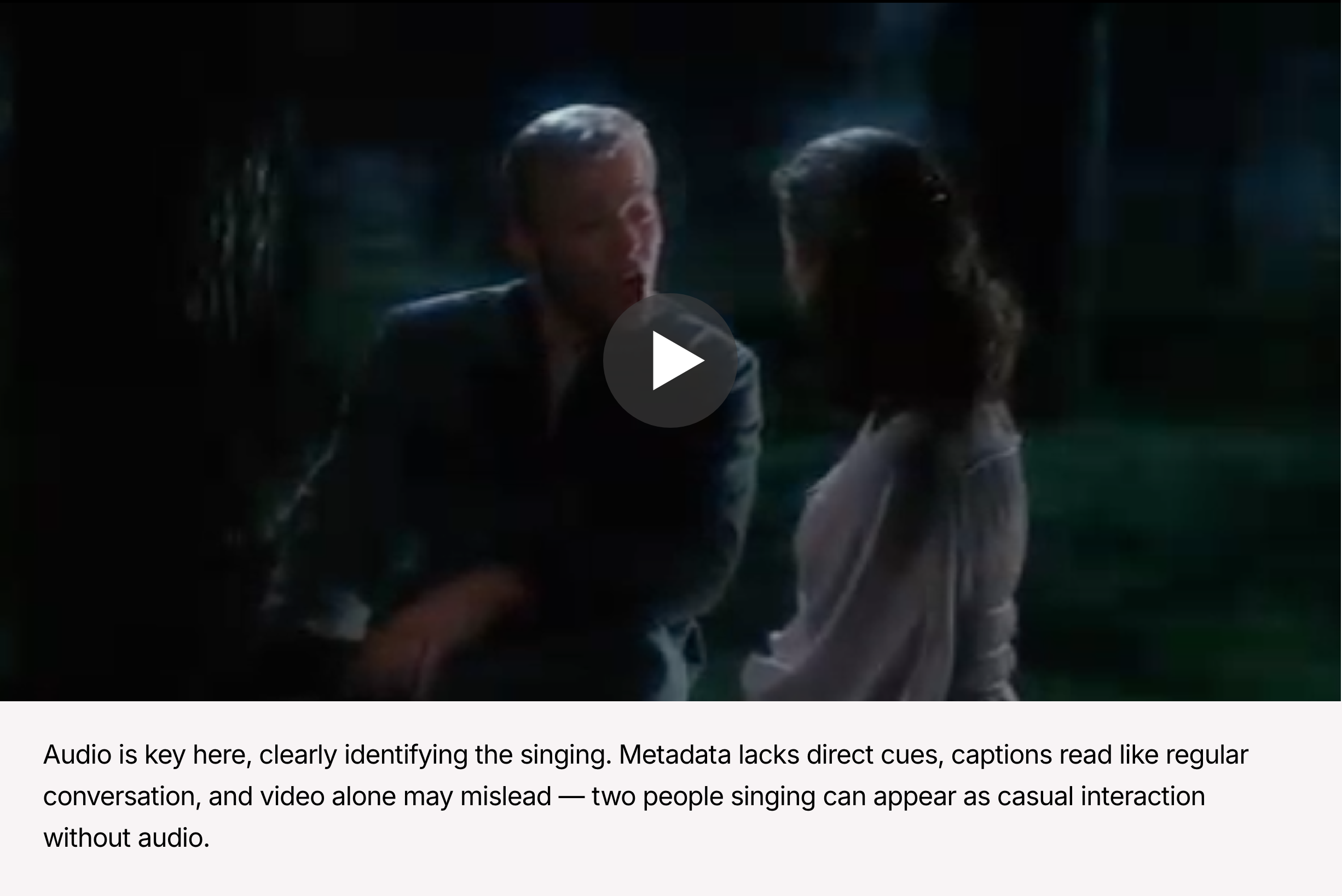
Video
Query: Chase scene from an animated movie
Retrieved Result by Video Modality:
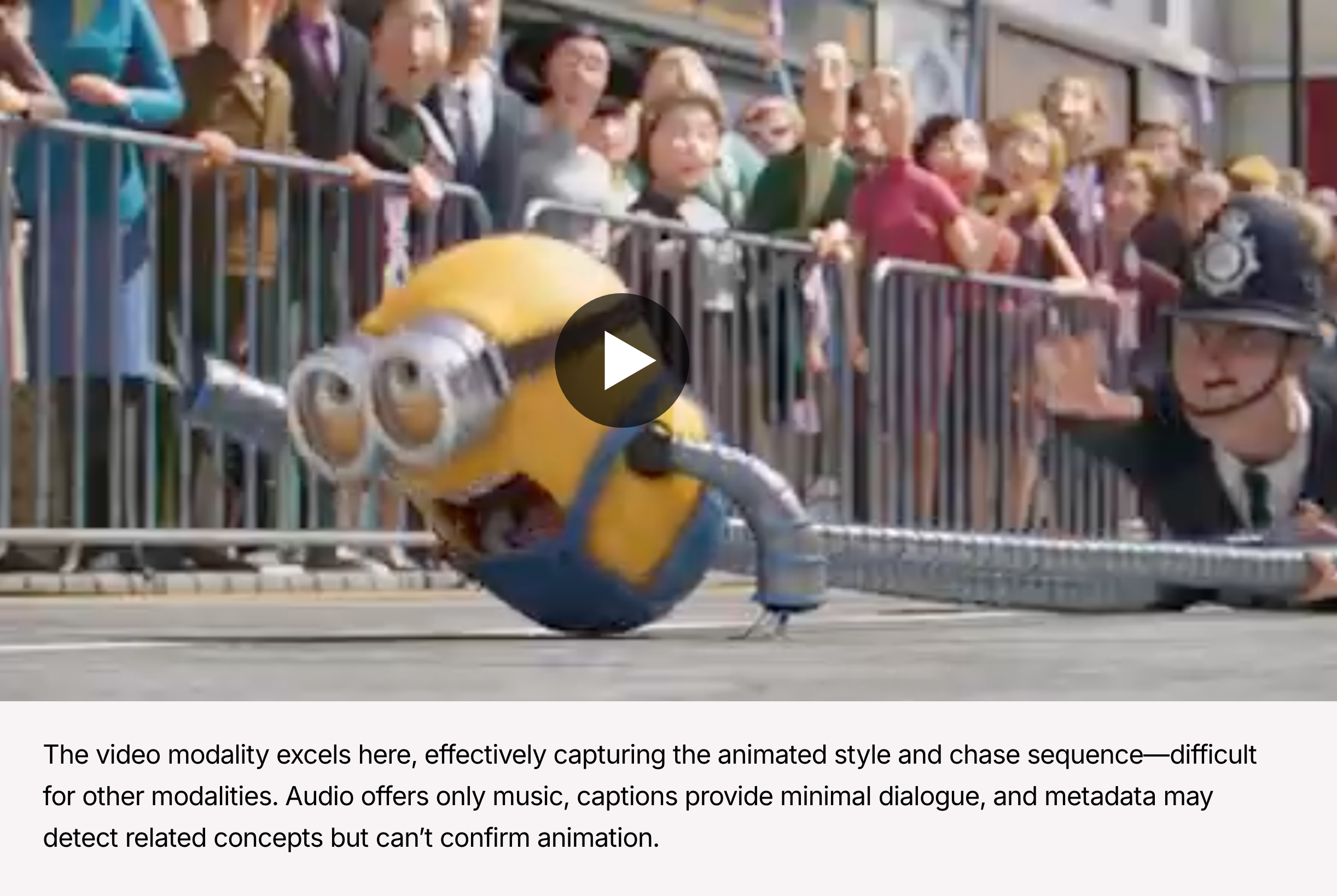