ContextIQ Benchmarking: Validation Datasets and Performance Metrics
Anoki Ai

We conducted a comprehensive evaluation of ContextIQ's efficacy by benchmarking it against state-of-the-art video retrieval models across publicly available datasets and benchmarks. We also address key evaluation challenges, particularly the differences between video domains in existing public datasets, such as shorter, amateur-produced content and the complex, high-production content typical of contextual video advertising. We created the Contextual Video Understanding (CVU) Dataset, a diverse collection of movie clips curated across a broad range of genres, production styles, and sources. Our datasets, retrieval queries and annotations are now publicly available at https://github.com/AnokiAI/ContextIQ-Paper.
Datasets
- MSR-VTT: We utilized the 1kA subset of the MSR-VTT test set, containing 1,000 videos, each paired with 20 textual descriptions. To address redundancy in textual descriptions (both within and across clips), we randomly sampled one caption per video for evaluation.
- Condensed Movies: Since the MSR-VTT dataset includes a variety of video rather than entertainment-focused content, we also utilize the Condensed Movies dataset. This dataset comprises scene clips from over 3,000 movies. We randomly selected 600 scene clips, extracting the first minute of each, and generated text queries focusing on concepts like objects, locations, emotions, and other contextual elements. Since the dataset lacks predefined tags for these queries, manual validation of results was conducted.
- Contextual Video Understanding (CVU): To evaluate ContextIQ's suitability for contextual ad targeting in the CTV landscape, we collected 500 movie clips from YouTube across diverse genres corresponding to advertisement categories (e.g., burger, concert, cooking, space shuttle, cowboys and western etc.). Each clip was annotated by at least two reviewers, with the union of their annotations used as ground truth.
MetricsWe reported the following metrics:
- Precision@K (P@K): Proportion of correct results among the top-K retrieved videos, averaged across all queries.
- Recall@K (R@K): Average number of queries for which at least one of the top-K retrieved videos is correct.
- Mean Average Precision@K (MAP@K): The mean of average precision scores at K across all queries.
Results
- MSR-VTT Evaluation:
Figure 1 compares ContextIQ's performance with Google’s Vertex AI. Despite not being jointly trained on multiple modalities, ContextIQ achieved comparable performance to Vertex AI and, in certain cases, outperformed it, highlighting its effectiveness in video understanding and retrieval.

- Condensed Movies Evaluation:
Figure 2 presents comparisons with TwelveLabs, a sophisticated multimodal model as well as LanguageBind. ContextIQ had similar performance as TwelveLabs and consistently outperformed LanguageBind across all metrics on the Condensed Movies dataset.
.png)
- CVU Evaluation:
Figure 3 illustrates ContextIQ’s performance advantages over baseline models, particularly at higher precision thresholds, where it consistently retrieves more relevant video content. While models such as Google Vertex, LanguageBind, and One-Peace benefit from joint multimodal training, ContextIQ’s expert-based and modular approach enables more precise retrieval by leveraging modality-specific embeddings. This targeted and multi-pronged approach allows ContextIQ to effectively capture fine-grained contextual information.
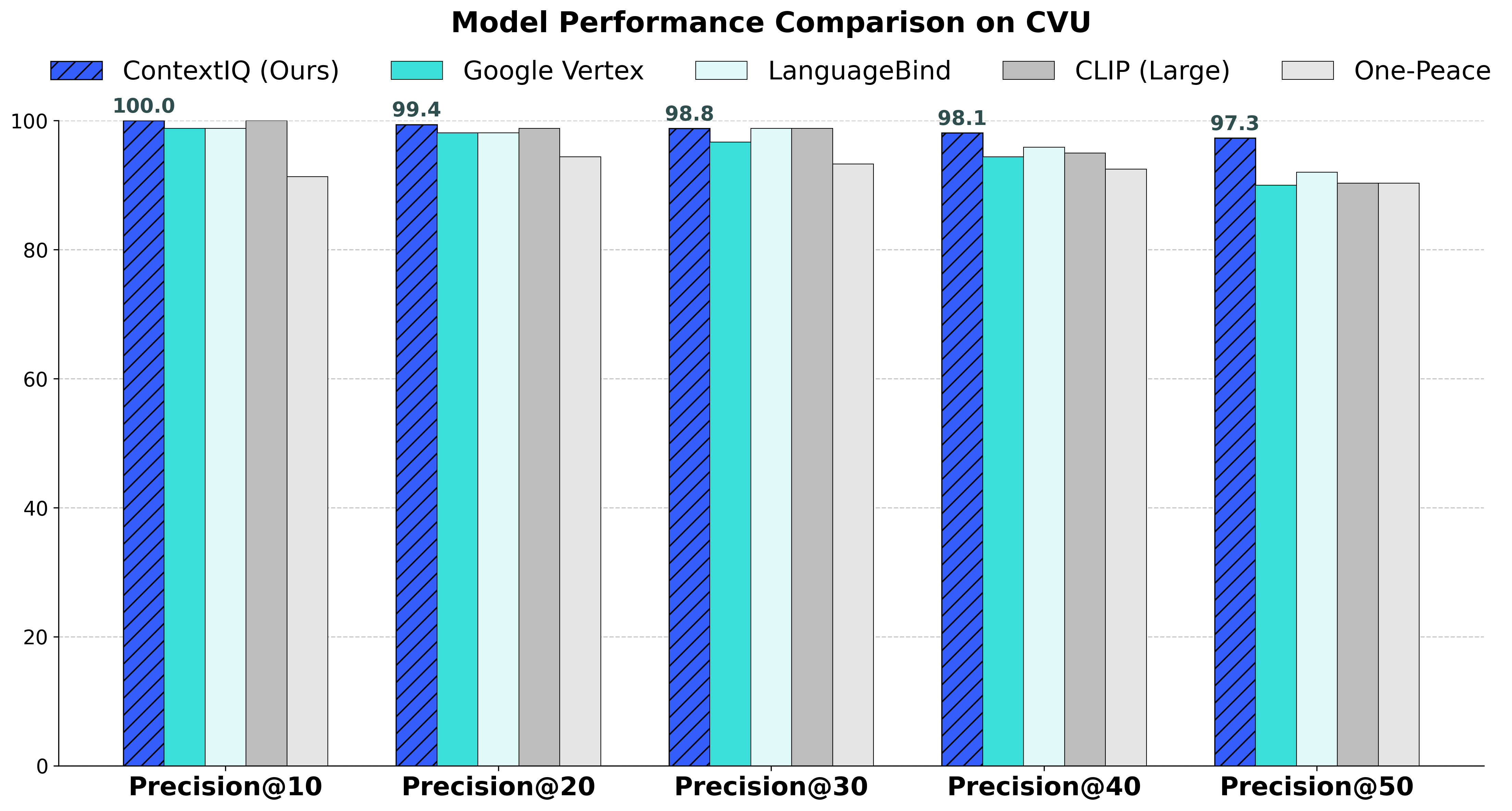
These findings demonstrate ContextIQ's robustness and applicability for tasks like contextual advertising and large-scale video retrieval. Leveraging its expert-model approach, ContextIQ consistently achieves performance comparable to, or exceeding, that of leading video retrieval systems across diverse datasets.
For additional details on the benchmark results, please refer to our paper.