ContextIQ: A Multimodal Video Understanding and Retrieval System for Contextual Advertising
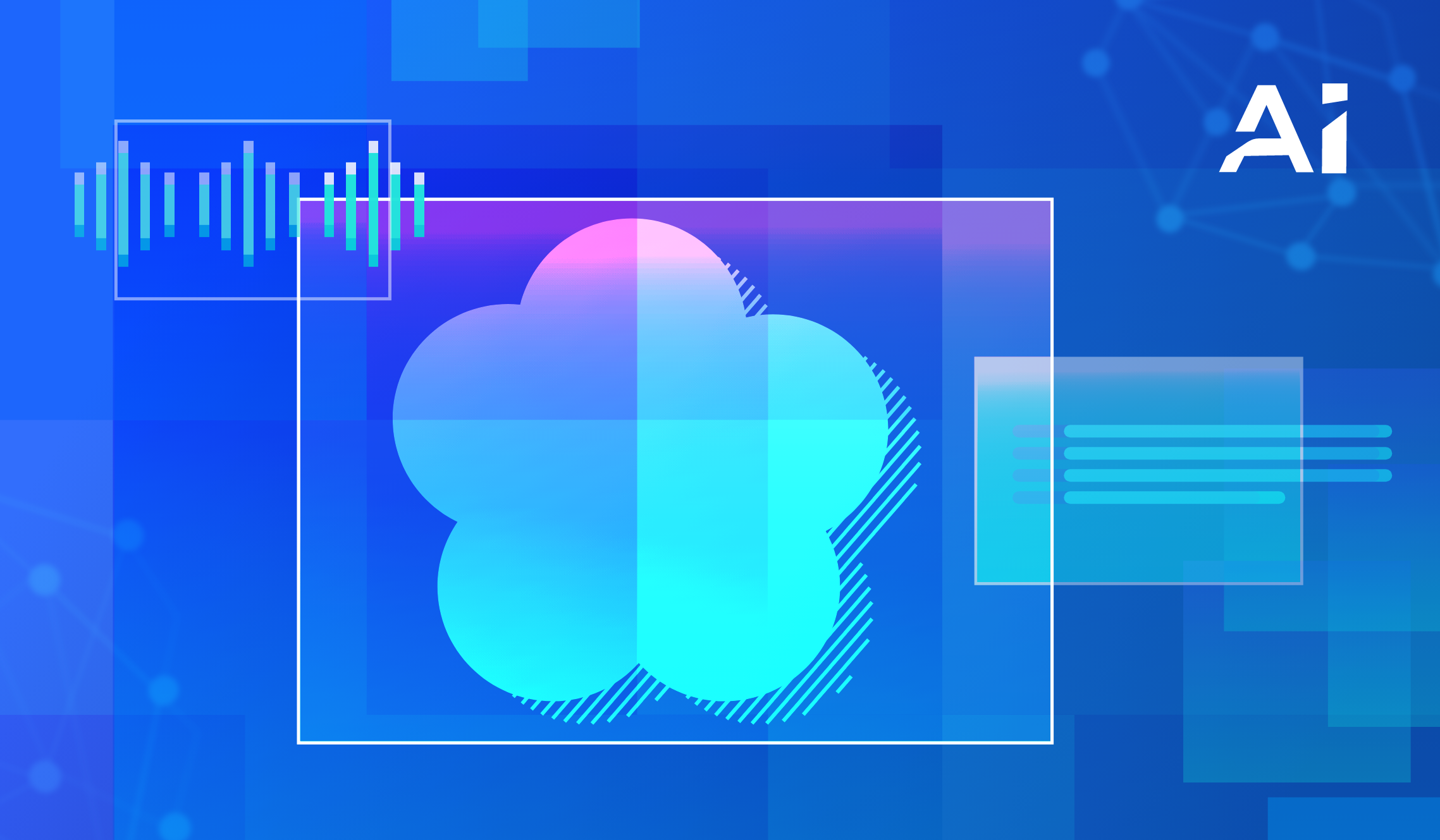
Contextual advertising aligns advertisements with the content being consumed, ensuring relevance and enhancing user engagement. This approach has long been established, with Google’s AdSense exemplifying its effectiveness in the context of web pages. However, with the rapid expansion of video content across social platforms and streaming services, extending contextual advertising to video content is the next logical step. At Anoki, we are pioneering this evolution by developing the first comprehensive, end-to-end contextual advertising system designed specifically for connected TV (CTV).
Modern video streaming platforms, with their vast content libraries, require advanced methods for analyzing video content to retrieve the most suitable content for ad placements for contextual advertising. To this effect we have built ContextIQ, a multimodal expert- based video understanding and retrieval system.
ContextIQ ingests long form contents and breaks it down into scenes that are typically 30-60 seconds, using a scene detection algorithm (Figure 1). ContextIQ is multimodal; meaning for each scene, it extracts signals across multiple modalities – video, audio, captions (transcripts), and metadata such as objects, places, actions, emotions, etc. to create semantically rich video representations. This helps in detailed understanding of scene level context just as a human would, allowing for precise ad placement and targeting.

In ContextIQ, information is extracted and stored as embeddings (Figure 1). Embeddings bridge the gap between artificial intelligence and human understanding by converting any media such as text/images/audio into a format/representation that machines can understand well. Which means its users are not restricted to a limited set of tags or keywords. A beauty brand can be interested in “scenes with women applying makeup” whereas a pet food company can search for “scenes with dogs” and an athletic wear brand could very well look at the same contents to find where the fitness related scenes are to place their ads. The possibilities are truly infinite.
Figure 2 illustrates the search pipeline for ContextIQ. Multi-modality allows for any-to-any search capabilities (text to video, audio to video, video to video and so on).

ContextIQ is the first ever end-to-end system with integration into ad serving systems (Figure 3). Depending on the brand campaign and the advertisements to be served, an advertiser defines a set of relevant queries. Using these brand-specific queries and the multimodal embeddings, ContextIQ’s multimodal search (Figure 2) identifies scenes where creatives can be contextually served. Additionally, these scenes can be passed through brand safety filters such as sentiment, profanity, hate speech, violence, substance abuse, and so on to ensure ads are placed within contextually appropriate and safe content.
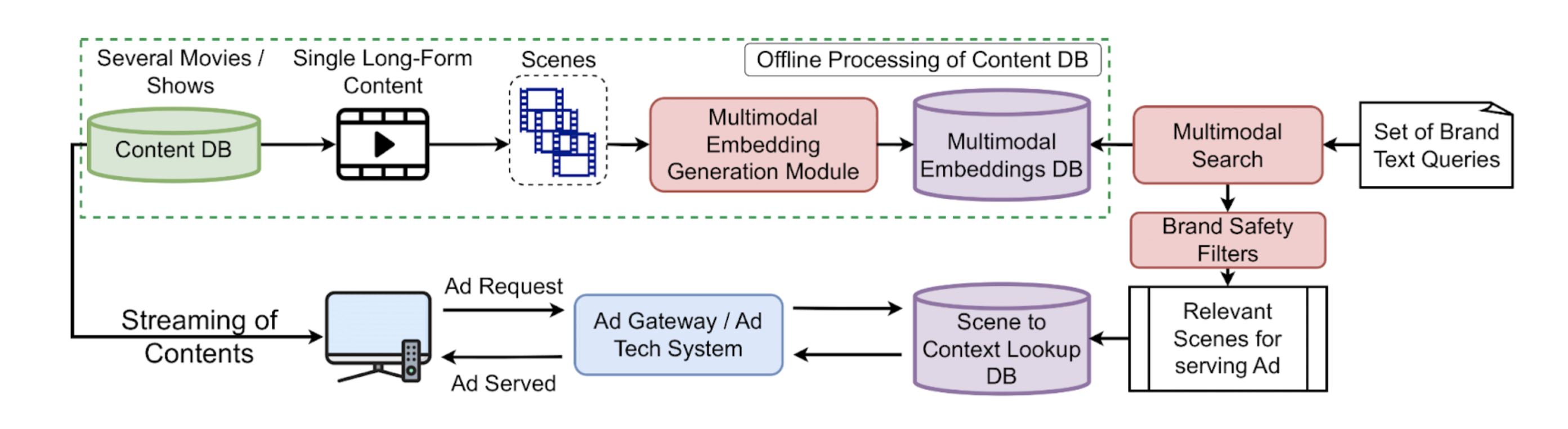
ContextIQ architecture is modular which provides us the flexibility to activate and/or search across only a subset of modalities if needed. This can lead to significantly faster indexing, enabling real time applications - in news channels for brand safety and sentiment filtering, for example. Additionally, each expert model can be fine-tuned to meet specific brand requirements with ease. For instance, for a beauty brand, we could integrate advanced object detection to spot specific niche beauty accessories.
Placing the right ad in the right context creates a seamless and pleasant ad viewing experience, resulting in higher audience engagement and, ultimately, better ad monetization. We at Anoki have built contextIQ, which does exactly that for video/CTV space. Please refer to our paper for more information and send us any comments to ml@anoki.tv.